The stage race was the Giant Crit Crushers series on Zwift. The data analyst was Fran Bambust. The pro-team was the sublime Roxsolt Attaquer pb Liv & SRAM, a UCI Women’s Continental Team with the likes of Peta Mullens (10th in the GC of the /Tour Down Under 2020) and Justine Barrow (2nd in the Australian National Championship 2020, selected for the UCI World Championship e-sports for Australia). The race of course had some other fabulous female racers as well, like Jenny Pettenon, Illi Gardner, Carlee Taylor, Courtney Sherwell, Bree Wilson, new talents Neve Bradbury, Sophie Sutton, Stephanie Corset and so on…
Fran has never raced outdoors. She has no titles, no pedigree, no UCI team and, with her 55 years of age, could be the mom of most of the other girls out on the track. What she did have though, was data and the data performance consultants from the company where she works.
Gauging the potential
First thing that data team did, of course, was looking at the potential and weaknesses of their Zwifting employee. They measured as minutely as possible of course, using not only the data of the trainer(a Tacx Smart Neo and a Kickr for comparison), Ultegra power cranks and Assioma Duo pedals. She was weighed and height measured and during this period checked by ZADA.
They gathered her data from Zwiftpower, Today’s Plan and TrainingPeaks, two data crunching applications for athletes, and analysed it using the insights of Andrew Coggan and Stephen McGregor*.
The analysts worked with these numbers and tried to build her up, not by doing workouts, but by racing the specific powers she lacked. Since her sprints seemed to be worst, they trained that most, which – although still low according to the Coggan insights – seemed to become her best power quite fast on Zwiftpower where she could compare to other Zwift Racers, being in the top 94% on w/kg and 98% in pure watts. Her 20 minutes power only put her in the 72% on wkg and 81% in watts.
Choosing the race
With that data the number crunchers could start looking for races that might suit her to exploit that power, without being hindered by her lower long range power. So we looked for short races, less than 20 minutes, that would probably end in a sprint. To heighten the probability of her ending near the podium they also figured they needed a series. More races would even out fabulous days and lesser days, hopefully getting her closer to the percentiles predicted on Zwiftpower.
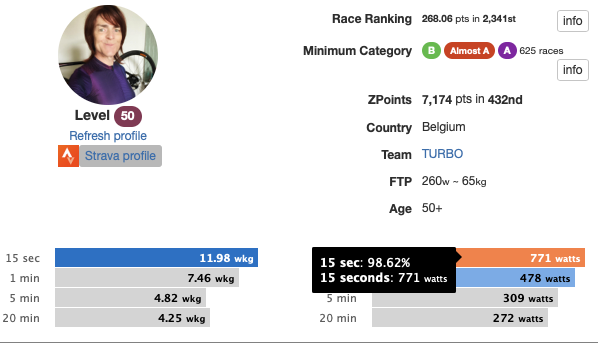
They also wanted to focus on the watts rather than the watts per kg – Fran being heavy compared to those young girls – and would thus look for a race series on a predominantly flatter terrain.
Understanding e-sports physics
Knowing those rules of physics of Zwift helps you racing in those online worlds. Quite often Zwift racers beat pro racers on Zwift because the latter have less experience with these rules. This would not be the case here. The RXS girls are extremely well versed in the laws of Zwift physics.
However, it was the data team’s hope that those ladies, like most women on Zwift, would overestimate the importance of watts per kg, and would try to put the hurt in the competition by putting out enormous w/kg.

On the flats however the pure watts would be more important than the watts per kg. So they would mainly be hurting themselves, since Fran’s heavier body allowed her to go for a higher wattage.
Fran would have to try and save as much energy as possible on the flats to be able to follow the light girls uphill on the few slopes the terrains would undoubtedly present. So Fran had to draft, staying in the slipstream of the others and conserving up to 30% of energy. On the occasional slope Fran would try to discourage attacks by semi-sprinting up herself, keeping the pace too high for others to fly away.
Knowing the competition and the courses
At the start of each race the data nerds would also analyse the powers of the competition. Who would have potentially attack where exactly? How should they use their team and personal potential on this course to their advantage? Figuring that out gave the data group an idea how to respond to that. They knew from the data that it would never be in Fran’s favour to make the race as hard as possible, since Fran would possibly be one of the first to perish from it. Anticipating to the stronger girls and sucking wheels till the finish would be the way to go.
That does sound simple, but it means you need to be sucking the right wheels. If the person you are drafting from drops a gap, you are gapped as well. So Fran would have to be sure to understand where those gaps would probably appear, and who would be the ones who would open a gap, or get dropped.
Of course you have to know as well when you need to start sprinting and from what position. To divine that again you have to know your data. How long can Fran hold her sprint and how many meters does that mean on this course: where should she start, and how long should she wait? And what does it mean if others sprint in front of you?
The data showed that you can use other racers to get slingshot around them if you time it right, and that you can sprint longer if you are on a lower gear/higher cadence before the sprint, and surf other sprinters using the draft during the sprint. The analysts also knew that waiting for the optical cue of other sprinters to start sprinting was wrong, because that optical cue has a delay: the racer has started sprinting even earlier than that. Fran had to stick to the plan and go where the team calculated she should.
Know the scoring systems, count the points
Last but not least the data group had to understand how the points were allocated. 20 points for the first one over the line, counting down from there. Ending up as high as possible in the first races was the first objective and from then on, we would start watching the competition. And: if you had no heart rate meter… you would not get any points at all. Which meant that, looking at the life data of the other racers, they could discard those without heart rate data, because those were only existing in terms of draft, but not as true competition. Fran would not have to chase those ladies.
Taking the lead in consistency
There were two stages a day and the data team soon noticed that Fran was weaker in the 2nd, that 2nd one counting over the 20 minute range. So they decided to try to gain points on the most important competition in the 1st stage, and try to hang on and control the gap on the 2nd stage. The competition noticed that as well after the 4th stage but by then Fran had a small advantage. Her results on the first 8 stages were: 2nd, 5th, 1st, 3rd, 2nd, 4th. At that point she had the lead in the race by ten points to Justine Barrow.
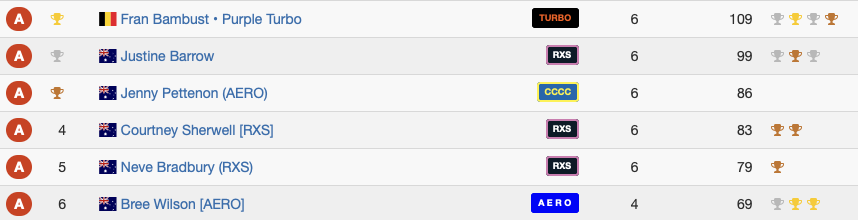
The finale
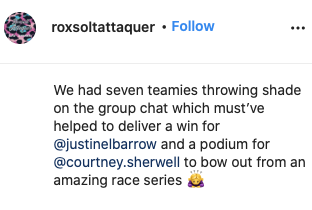
With two races to go, there were 40 points left to win, meaning Bree Wilson could still come up to Fran’s position if Fran got absolutely nothing… which could have actually happened since Fran managed to fall during exercise breaking both her trainer and her bike. Fortunately she managed to replace both in time for the race.
More realistically, the RXS ladies would play Justine’s card and not only use her as a decoy for Courtney or Neve. That would have been too great a risk. The data team figured RXS would send in as many team members as possible and try to get them between Justine and Fran. The main objective was to let Justine and Jenny, nrs 2 and 3 in the GC, take as little points as possible on Fran while saving enough energy for race 2.
Stage 7. Penultimate stage. The RXS girls put the hamer down from the start of stage 7, but Fran got the perfect assistance of AHDR/The Purple Armada team members Katherine Mackay, Sam Smagas, Marylou Fitz, Julia Broom and Justine Clark to keep the group together in the first laps.
Watching data during stage 7. Fran’s heart rate looks quite ‘relaxed’, not in overdrive. Something wrong with Carlee’s heart or meter though. Annabel will not get points. The 5 second gap at that point was nothing to worry about, as Fran still had Justine C and Kath M nearby and Justine B by her side.
Fran was too far back in the group at the time of the sprint and started sprinting too late, but still managed to finish behind Justine who finished 2nd. Justine had closed in with one point.
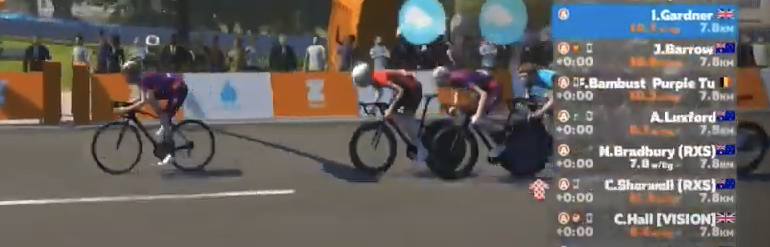
Stage 8. Final stage.A 9 points gap when there are only 20 points available may seem like a comfortable gap but nothing was further from the truth. The last stage offering a very hilly course the odds were very much against Fran. The finish was at the top of a 900 meter climb which meant the race would be decided with w/kg instead of pure watts. More than ever Fran had to be vigilant, because RXS would try to force a breakaway hoping to get a group of more than 9 people in, preferably without Fran.
That breakaway did form… But with the help of her teammate Justine C, Fran managed to bridge to that breakaway. Still not safe though, because that breakaway had 12 people in them and Justine B would quite probably be the strongest of that group in the uphill finish. Fran had to make sure not to end up off the back or on the tail. So whenever she dropped a bit on the climbs, she had to go deep to get back in there. And she did. She was at the rear end of that breakaway group at the start of last climb, but started overtaking ladies one by one going for that finish, dropping the sprint at 300 meter to go…
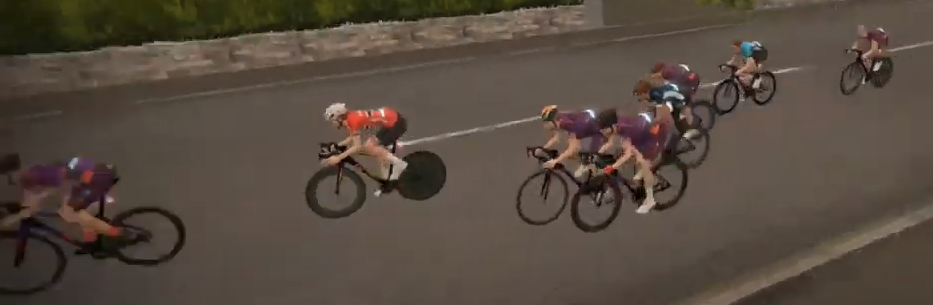
Justine Barrow did win, ahead of her team mate Courtney Sherwell, who must have hoped 7 more random ladies would be behind her. But Fran was there. Taking third, taking the overall win.
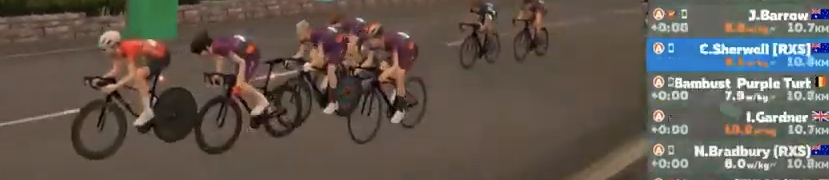
Data for the win?
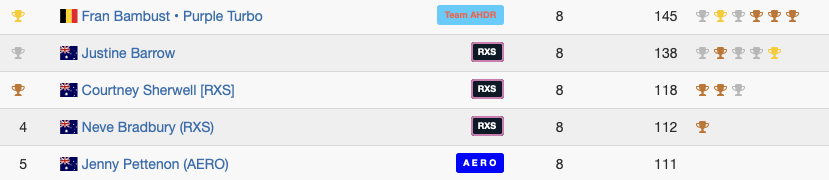
Did Fran only win because of the data? Of course not. She had to train as well. But the data did help them pick the right arena for her to win. Data can help you to pick your battles, point out the tipping points, gauge your opponents and take the most effective decision towards your goal, given your strengths and weaknesses and that of your opponents. And yes, you can defeat the bigger party.
*Allen, H. Coggan A(2010) Training and racing with a power meter. Chicago
This article appeared first on theda.ai, the website of The Data Arena
#DataArena